Deep Learning Vs. Machine Learning- Difference Between
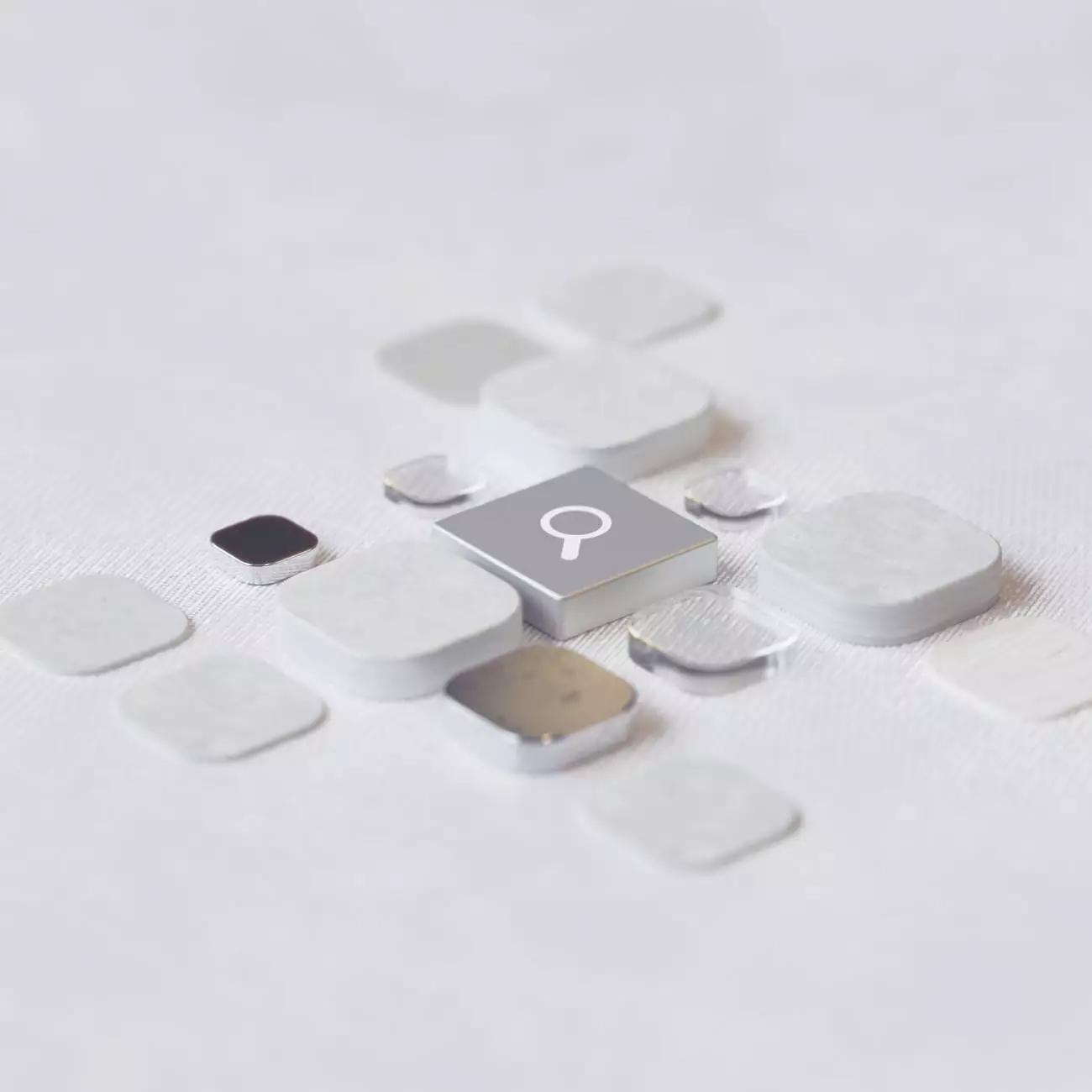
Welcome to Maslow Lumia Bartorillo Advertising's insightful page on the key differences between Deep Learning and Machine Learning. In the rapidly evolving field of artificial intelligence (AI) and data science, understanding the distinctions between these two methodologies is crucial for businesses and organizations striving to harness the power of intelligent technologies to drive informed decision-making and competitive advantage.
The Rise of Machine Learning
Machine Learning, a subset of AI, focuses on algorithms and statistical models that enable computer systems to automatically learn and improve from experience without explicit programming. It involves the development of models capable of analyzing large datasets, identifying patterns, and making predictions or decisions based on the data.
Businesses across various industries have embraced Machine Learning due to its versatility and effectiveness. From recommendation engines to fraud detection systems, Machine Learning has proven its value in automating tasks, improving operational efficiency, and unlocking valuable insights hidden within data.
The Emergence of Deep Learning
Deep Learning, on the other hand, is a specific subfield of Machine Learning that emphasizes the use of artificial neural networks and deep neural networks inspired by the human brain's structure and functioning. It aims to simulate the way humans process and analyze information, enabling machines to understand data through a hierarchical learning approach.
Deep Learning has gained significant attention and achieved remarkable breakthroughs in recent years, particularly in the areas of computer vision, natural language processing, and voice recognition. It has powered advancements like self-driving cars, image recognition, and natural language understanding, revolutionizing several industries and challenging traditional problem-solving techniques.
Key Differences Between Deep Learning and Machine Learning
1. Complexity and Interpretability
One of the primary differences between Deep Learning and Machine Learning lies in complexity and interpretability. Machine Learning algorithms can provide transparent and interpretable models, allowing humans to understand the reasoning behind the predictions or decisions. In contrast, the inner workings of Deep Learning models, constructed with multiple layers of interconnected neurons, often lack interpretability, making it challenging to explain their outputs.
2. Data Requirements
Machine Learning models typically require labeled datasets for training, where each data point is associated with a known outcome. These models rely on feature engineering, where domain experts select and define relevant features to train the algorithm effectively.
Deep Learning models, on the other hand, excel in learning from raw, unstructured data, such as images, text, and speech. They can automatically extract features from the input data, eliminating the need for manual feature engineering. However, Deep Learning models often demand large amounts of labeled data for training and can suffer from overfitting if the dataset is limited.
3. Computation Power
Deep Learning techniques heavily rely on complex neural network architectures, which demand significant computational resources to train effectively. Training a Deep Learning model typically involves training thousands or millions of parameters, requiring high-performance GPUs or specialized hardware accelerators.
Machine Learning algorithms, by comparison, are often less computationally intensive and can be trained on standard hardware configurations. This difference in computational requirements makes Deep Learning a more resource-intensive approach, necessitating careful consideration of the available infrastructure and associated costs.
4. Feature Extraction
Deep Learning models have the advantage of automatically learning relevant features through their layered structures. They can discover intricate patterns and representations from the raw data, making them suitable for complex tasks like image classification or speech recognition.
Machine Learning models, on the other hand, require manual feature engineering, where domain knowledge and expertise are crucial in selecting and representing meaningful features. The effectiveness of a Machine Learning model heavily depends on the quality of the chosen features.
5. Training Time
Deep Learning models often require substantial training time, especially when dealing with large datasets or complex problems. The iterative nature of training deep neural networks can be time-consuming, requiring extensive computational resources and optimal parameter tuning.
Machine Learning algorithms generally have shorter training times compared to Deep Learning models. They are often faster to train, making them more suitable for scenarios where real-time or near-real-time decision-making is necessary.
Choosing the Right Approach for Your Business
Both Deep Learning and Machine Learning offer unique advantages and have their respective strengths in different domains. When deciding on the most suitable approach for your business, it's vital to consider various factors, such as the nature of the problem, available data, computational resources, interpretability requirements, and the desired level of complexity.
If your business involves tasks like image recognition, natural language processing, or voice-based applications, Deep Learning may be the ideal choice. Its ability to learn from raw, unstructured data and extract high-level features can provide outstanding results.
On the other hand, if explainability and transparency are paramount, or if you have limited labeled data, Machine Learning may be a more viable option. Traditional Machine Learning techniques can deliver interpretable models and can be effective when dealing with structured or labeled data.
It's worth noting that a hybrid approach, combining elements of Deep Learning and Machine Learning, can also be beneficial in certain scenarios. This fusion of methodologies allows businesses to leverage the strengths of both approaches and create innovative AI solutions that address complex challenges.
Final Thoughts
Deep Learning and Machine Learning are powerful tools within the AI landscape, each with its own unique characteristics and applications. Understanding the differences between the two is vital in determining the right approach for your organization's specific needs and objectives. At Maslow Lumia Bartorillo Advertising, a leading provider of innovative marketing and advertising solutions, we understand the intricacies of these technologies and can help you navigate the fast-paced world of AI. Contact us today to discuss how we can assist you in harnessing the potential of Deep Learning, Machine Learning, and other cutting-edge AI technologies to drive your business forward.