Unlocking the Future: The Role of Medical Image Annotation in Healthcare
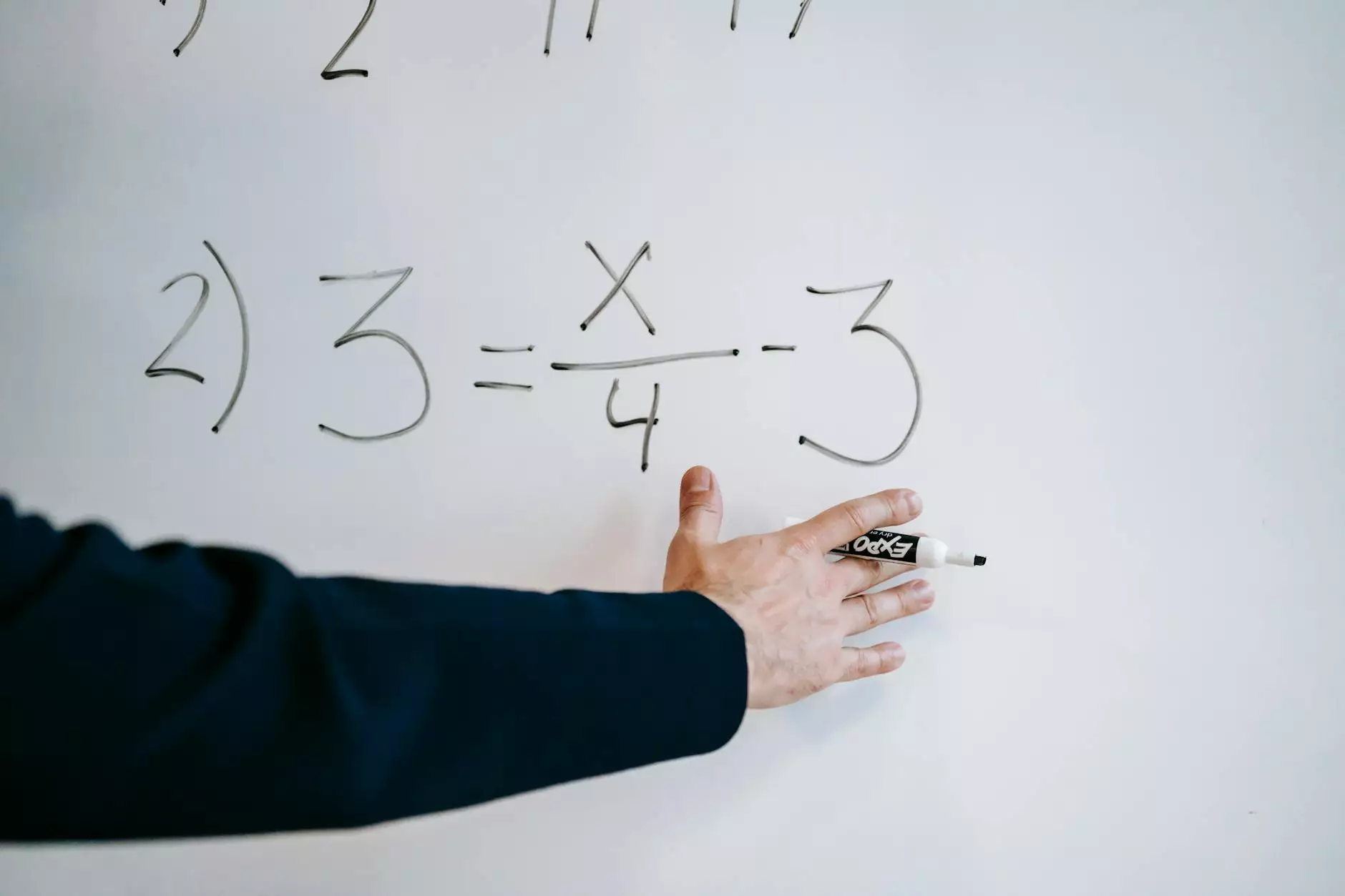
The healthcare industry has experienced a revolution with the advent of advanced technologies, particularly in the realm of diagnostics. One of the most significant innovations is medical image annotation, a process that transforms how medical professionals interpret imaging data. As we delve into the intricacies of medical image annotation, we'll explore its fundamental importance in diagnostics, the technologies that enable it, and its overall impact on patient care.
Understanding Medical Image Annotation
Medical image annotation refers to the process of labeling or tagging images acquired through various imaging modalities, such as MRI, CT scans, and X-rays. The primary goal is to create a structured dataset that can be utilized for training machine learning models to assist in diagnosis and treatment planning.
Types of Medical Imaging
Before discussing the annotation process, it is essential to understand the types of medical imaging that play a critical role in patient diagnostics:
- X-rays: Used to visualize the internal structure of the body, particularly bones.
- CT Scans: Provide detailed cross-sectional images of the body.
- MRI: Utilizes magnetic fields to produce detailed images of organs and tissues.
- Ultrasound: Uses sound waves to create images of the inside of the body.
- Pet Scans: Detects metabolic activity and is commonly used in oncology.
The Importance of Medical Image Annotation
In the modern healthcare environment, medical image annotation has become indispensable for several reasons:
1. Enhancing Diagnostic Accuracy
Accurate diagnosis is the cornerstone of effective treatment. Medical image annotation improves the consistency and reliability of diagnostic results by providing a standardized method for interpreting images. Annotated images help radiologists identify anomalies more efficiently, thereby improving the chances of early detection of diseases such as cancer.
2. Facilitating Machine Learning and AI Integration
The healthcare industry is increasingly leveraging artificial intelligence (AI) to assist in diagnostics. Well-annotated datasets are crucial for training machine learning models. These models learn to recognize patterns and make predictions based on annotated images, thus aiding healthcare providers in making more informed decisions.
3. Streamlining Clinical Workflows
As healthcare providers face mounting pressure to deliver timely diagnoses, medical image annotation helps streamline workflows. Automated annotation tools can significantly reduce the time required to analyze images, allowing radiologists to focus on critical cases and improving overall patient care.
Technologies Used in Medical Image Annotation
Various technologies play essential roles in the medical image annotation process. Understanding these will help elucidate how the field is evolving:
1. Deep Learning Algorithms
Deep learning techniques, particularly convolutional neural networks (CNNs), are at the forefront of medical image annotation. These algorithms are designed to mimic the human brain's ability to recognize patterns and can significantly enhance the accuracy of image interpretation.
2. Annotation Tools and Software
A variety of annotation tools are available that help medical professionals annotate images quickly and efficiently. Popular tools include: - Labelbox: A collaborative platform for annotating images and managing datasets. - VGG Image Annotator (VIA): An open-source tool that provides a simple interface for image annotations. - DeepZoom: A tool tailored for medical applications to facilitate the annotation of whole slide images.
3. Cloud Computing
Cloud technology has revolutionized how data is stored and accessed. By leveraging cloud resources, healthcare providers can store vast amounts of annotated images, ensuring accessibility and security while also facilitating collaboration among medical teams across different locations.
Challenges in Medical Image Annotation
Despite its advantages, the process of medical image annotation is not without challenges, including:
1. Variability of Images
Medical images can vary significantly in quality and style depending on the imaging device used. This variability can complicate the annotation process and affect the accuracy of AI models trained on inconsistent data.
2. Time-Consuming Process
Manual annotation can be highly labor-intensive and time-consuming, especially when dealing with large datasets. Automation technologies are being developed to help mitigate this issue, but they require carefully curated datasets to perform effectively.
3. Need for Expert Annotations
For high accuracy, annotations must be performed by experts. This requirement can create a bottleneck in the annotation process, as qualified radiologists may have limited availability to perform this critical task.
Future Prospects of Medical Image Annotation in Healthcare
The future of medical image annotation looks promising, with several trends poised to shape its evolution:
1. Increased Use of Artificial Intelligence
As AI technology continues to evolve, its integration into medical image annotation processes will likely become even more pronounced. AI algorithms will automate much of the annotation work, leading to faster procedures and potentially freeing up radiologists for more complex tasks.
2. Enhanced Collaboration Among Healthcare Professionals
Cloud-based annotation tools will facilitate collaboration among multidisciplinary teams. This collaboration will ensure a more holistic approach to patient care, as specialists can share insights and annotations from different geographic locations.
3. Improved Patient Outcomes
As medical image annotation becomes quicker and more reliable through technological advancements, patient outcomes will improve. Early detection and diagnosis will become more achievable, ultimately contributing to better patient management and treatment strategies.
Conclusion
In conclusion, medical image annotation represents a pivotal advancement in the healthcare sector. By enhancing diagnostic accuracy, facilitating AI integration, and streamlining clinical workflows, this process has the potential to drastically change how healthcare is delivered. Despite challenges such as the need for expert annotations and variability in images, the future prospects for medical image annotation remain bright. As technology continues to advance, its role in improving patient outcomes and revolutionizing healthcare services will only grow stronger.
As experts in the field, it’s crucial for healthcare providers to stay updated on the latest trends in medical image annotation to leverage its full potential. By prioritizing this essential component of medical imaging, the industry can pave the way for innovation, improved diagnostics, and ultimately enhanced patient care.