Unlocking Insights: The Role of Healthcare Datasets for Machine Learning in Business
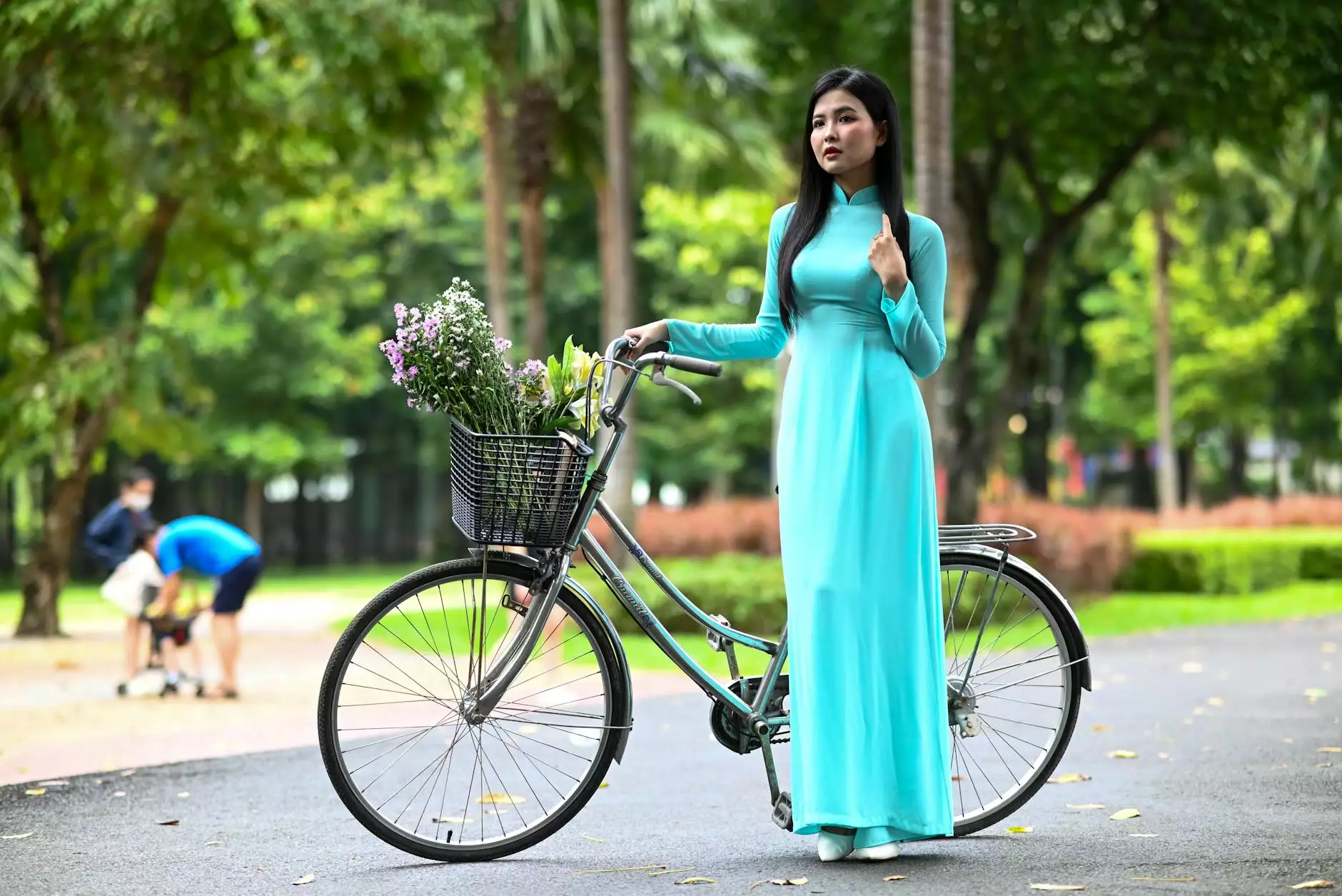
In today’s data-driven world, the healthcare industry is undergoing a transformation powered by machine learning and artificial intelligence. The healthcare datasets for machine learning have become a valuable resource for businesses aiming to enhance their services, optimize operations, and improve patient outcomes. This article explores how these datasets can be leveraged to create significant advantages in the healthcare sector, focusing on various applications, the importance of data quality, and how businesses can effectively utilize these datasets.
Understanding Healthcare Datasets
Healthcare datasets refer to the structured collections of data that capture patient information, clinical outcomes, treatment histories, and more. These datasets are essential for conducting research, analyzing trends, and developing machine learning models that can predict and improve patient care.
Types of Healthcare Datasets
Healthcare datasets can be categorized into several types:
- Electronic Health Records (EHRs): Comprehensive patient records that include data on patient demographics, medical history, medication, and treatment plans.
- Clinical Trial Data: Information collected from studies conducted to evaluate new drugs, treatments, or medical devices.
- Claims Data: Information generated from insurance claims that provide insights into patient healthcare utilization and costs.
- Genomic Data: Data pertaining to an individual’s genetic makeup, which can help personalize treatment plans and predict health risks.
- Wearable Device Data: Information collected from fitness trackers and other health monitors that track physical activity, heart rate, sleep patterns, and more.
The Impact of Machine Learning on Healthcare
Machine learning has the potential to transform healthcare by making predictions, personalizations, and automations that were previously unimaginable. With the integration of healthcare datasets for machine learning, organizations can make data-backed decisions that improve patient care and operational efficiency.
Predictive Analytics in Patient Care
One of the most significant applications of healthcare datasets in machine learning is predictive analytics. By analyzing historical patient data, machine learning algorithms can identify patterns that help predict patient outcomes. For example, predictive models can forecast diseases, patient readmissions, or the likelihood of complications during treatment.
Personalized Treatment Plans
Using healthcare datasets for machine learning, practitioners can develop personalized treatment plans tailored to individual patient data. This approach enhances treatment efficacy and minimizes the risk of adverse reactions. By analyzing genomic data alongside traditional medical records, healthcare professionals can create customized therapies that target specific patient profiles.
Operational Efficiency
Businesses in the healthcare sector are leveraging machine learning to streamline operational processes. Machine learning algorithms can analyze patient flow data to optimize staffing, resource allocation, and scheduling. This leads to improved service delivery and reduced wait times for patients.
Data Quality: The Cornerstone of Effective Machine Learning
The effectiveness of machine learning models is heavily dependent on the quality of data used. High-quality healthcare datasets for machine learning must be accurate, complete, and representative of the population. Poor data quality can lead to erroneous conclusions and misinformed decisions, undermining the potential benefits of machine learning in healthcare.
Ensuring Data Integrity
Several best practices can be implemented to ensure the integrity of healthcare datasets:
- Data Cleaning: Regularly updating and correcting data to eliminate inaccuracies.
- Data Standardization: Utilizing consistent formats for capturing and storing data to enhance interoperability.
- Data Validation: Implementing protocols to regularly check data for completeness and reliability.
Challenges in Utilizing Healthcare Datasets
While the benefits of healthcare datasets are substantial, there are also challenges that businesses must navigate. Key challenges include:
Privacy and Security Concerns
Healthcare data is sensitive and must be managed with the utmost care. Organizations must comply with regulations such as HIPAA in the U.S. to protect patient information. Ensuring data privacy requires robust security measures, including encryption, access controls, and regular audits.
Data Silos
In many healthcare organizations, data is stored in silos, making it difficult to access comprehensive datasets. Breaking down these silos to create a unified dataset is crucial for effective machine learning applications.
Interoperability Issues
Diverse data formats and systems hinder seamless integration and analysis. Industry-wide standards are essential for improving interoperability among healthcare systems and making data sharing more efficient.
Best Practices for Businesses Using Healthcare Datasets
To successfully utilize healthcare datasets for machine learning, businesses should adopt the following best practices:
Invest in Advanced Analytics Tools
Employing sophisticated analytics tools allows healthcare organizations to glean insights from large datasets efficiently. Machine learning platforms can automate data processing, enabling quicker analysis and decision-making.
Collaborate with Data Experts
Partnering with data scientists and machine learning experts can significantly enhance an organization’s ability to extract meaningful insights from healthcare datasets. Collaborations can lead to innovative applications of machine learning in various healthcare operations.
Prioritize Data Governance
Establishing strong data governance protocols ensures that data is managed responsibly and ethically. This includes defining roles and responsibilities for data management and establishing frameworks for data usage that comply with legal standards.
Case Studies: Successful Implementations of Machine Learning in Healthcare
Several healthcare organizations have successfully implemented machine learning techniques using robust healthcare datasets, resulting in improved patient care and operational efficiencies:
1. Predictive Readmission Models
Several hospitals have utilized machine learning algorithms to develop predictive models for patient readmissions. By analyzing EHR data, these models can identify at-risk patients, allowing healthcare providers to implement targeted interventions that reduce readmission rates and improve patient outcomes.
2. Drug Discovery and Development
Pharmaceutical companies are leveraging machine learning to accelerate the drug discovery process. By analyzing genomic datasets and clinical trial data, companies can identify potential drug candidates much faster than traditional methods, leading to quicker development times and reduced costs.
3. Operational Analytics for Resource Management
Healthcare systems are increasingly using machine learning for operational analytics. For example, predictive modeling can optimize staffing levels and enhance patient flow management, ultimately leading to improved efficiency and reduced wait times.
Conclusion: Embracing the Future of Healthcare with Machine Learning
The integration of healthcare datasets for machine learning presents immense opportunities for innovation and improvement in the healthcare sector. By harnessing these datasets, businesses can drive better patient outcomes, enhance operational efficiencies, and stay ahead in a competitive industry. As the healthcare landscape continues to evolve, organizations that embrace these technologies will be well-positioned for success.