Unlocking the Potential of Data Labeling Platforms
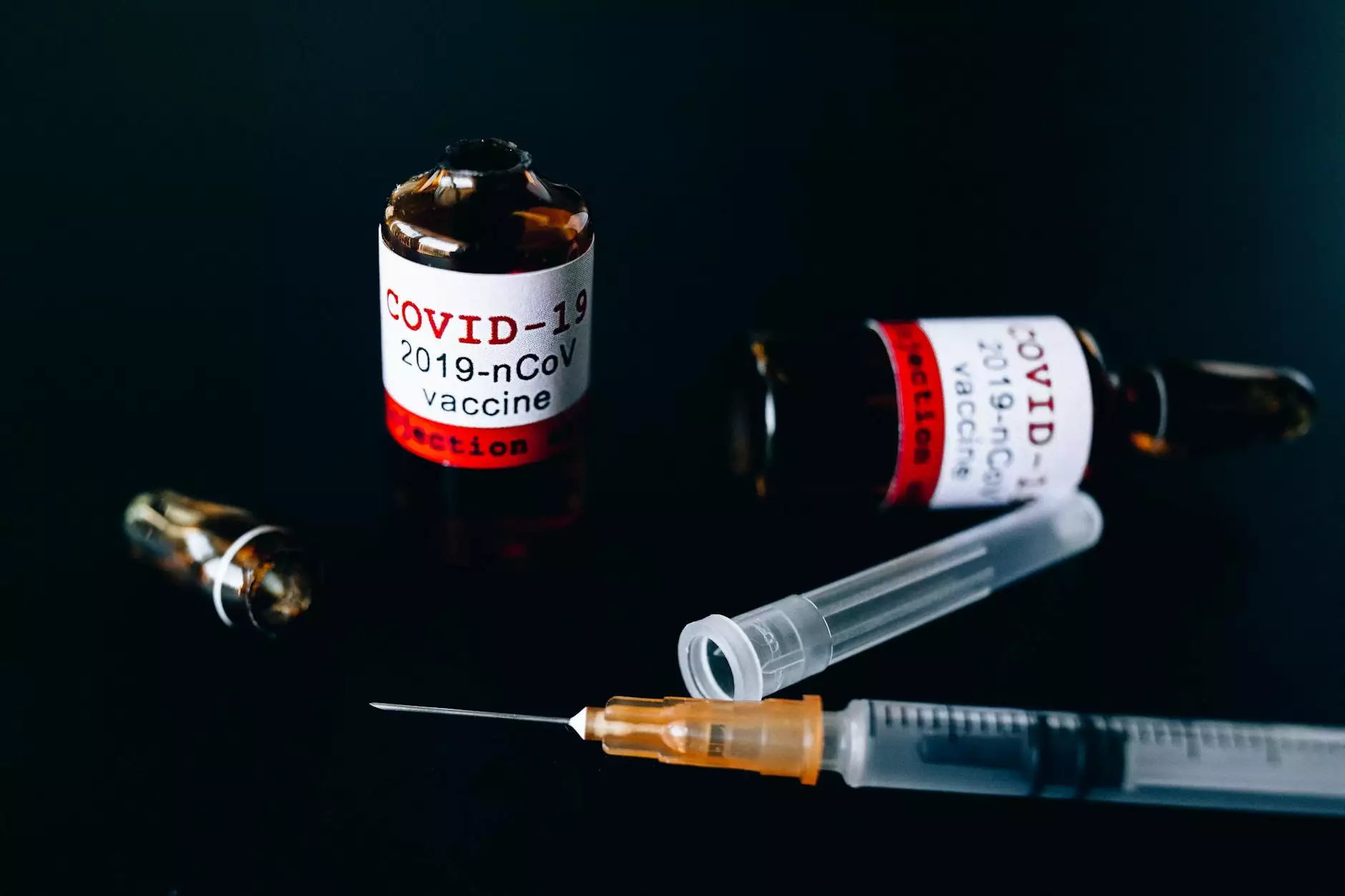
The surge in artificial intelligence (AI) and machine learning (ML) applications has significantly increased the demand for robust data preparation solutions. A data labeling platform is at the forefront of this transformation, playing a critical role in ensuring that AI algorithms are trained effectively and efficiently. In this article, we will explore the various aspects, functionalities, and benefits of utilizing a data labeling platform to enhance your AI projects, particularly focusing on offerings from Keylabs.ai.
Understanding Data Labeling
Before delving into the specifics of a data labeling platform, it’s essential to understand what data labeling entails. Data labeling is the process of annotating data—be it images, text, or audio—so that AI models can comprehend and learn from it. The labels serve as the backbone of machine learning algorithms, allowing them to recognize patterns and make predictions. For instance, in image recognition, labeling involves identifying objects within images, which helps in training models to recognize those objects in new images.
The Importance of Data Labeling
Data labeling is pivotal in various applications, including:
- Computer Vision: Training models to detect and classify objects within images.
- Natural Language Processing: Identifying sentiment, entities, and context in textual data.
- Speech Recognition: Transcribing and interpreting spoken words into text.
- Medical Diagnosis: Assisting in identifying and diagnosing conditions from medical imagery.
What is a Data Labeling Platform?
A data labeling platform is a sophisticated tool designed to streamline the data annotation process. These platforms provide a user-friendly interface, enabling annotators—be it data scientists, trained professionals, or even crowdsourced individuals—to label vast datasets efficiently. These platforms often come equipped with various features tailored to enhance productivity, accuracy, and speed in data labeling tasks.
Key Features of a Data Labeling Platform
When considering a data labeling platform, it is essential to look for specific features that can maximize your team's productivity and the quality of your labeled data:
- Customizable Annotation Tools: Platforms should allow customization to cater to the specific needs of your dataset, whether it involves bounding boxes for image labeling or entity recognition in text.
- Collaboration Features: Functionality for multiple users to work simultaneously, allowing for greater flexibility and faster project completion.
- Quality Assurance Mechanisms: Built-in tools to verify and validate the accuracy of the annotations, ensuring high data quality.
- Integration Capabilities: Seamless integration with existing data pipelines and other tools used in AI development.
- Analytics and Reporting: Tools to monitor progress and evaluate the efficiency of annotation efforts through detailed reporting.
Benefits of Using a Data Labeling Platform
Utilizing a data labeling platform can lead to numerous advantages for organizations engaged in AI and ML development. Here are some key benefits:
1. Increased Efficiency
Manual data labeling can be a labor-intensive and time-consuming process. A dedicated platform accelerates this process through optimized workflows, automated tools, and an intuitive interface. This enables teams to annotate larger datasets in significantly less time, facilitating faster deployment of AI models.
2. Enhanced Accuracy
By employing built-in quality control measures and validation processes, data labeling platforms help achieve higher accuracy levels in annotations. This is crucial, as the success of AI models heavily relies on the quality of the training data.
3. Cost-Effective Solutions
Outsourcing data labeling may lead to significant costs. Implementing an efficient data labeling platform can help manage internal resources more effectively, reducing the need for extensive external services and lowering overall project costs.
4. Scalability
A data labeling platform supports scalability, allowing organizations to handle increasing volumes of data effortlessly. As your AI requirements grow, these platforms can adapt to meet the rising demand for labeled data without compromising on speed or quality.
5. Access to Expertise
Many platforms provide access to a community of expert annotators who specialize in various fields. This means your data will be labeled accurately, utilizing professionals familiar with the specific nuances of your industry.
Choosing the Right Data Labeling Platform
With numerous options available in the market, selecting the ideal data labeling platform can be overwhelming. Here are some essential considerations:
1. Understand Your Requirements
Before diving into the market, clearly outline your project requirements. Consider the types of data you will be working with, the volume of data, and your budget constraints. This clarity will help you filter platforms that best suit your needs.
2. Evaluate the Platform’s Features
Ensure the platform you choose has the essential features discussed earlier. Pay special attention to collaborative tools, customization options, and integration capabilities, as these will greatly influence your annotation workflow.
3. Check for Support and Resources
A reliable data labeling platform should offer robust customer support and resources such as tutorials, webinars, and documentation. This support can be invaluable in ensuring that your team can leverage the platform to its fullest potential.
4. Read Reviews and Testimonials
Research user reviews and case studies to gain insights into real-world applications of the platform. User feedback can provide a clear picture of strengths and weaknesses, helping you make a more informed decision.
Case Studies: How Companies Leverage Data Labeling Platforms
To illustrate the impact of data labeling platforms, let's delve into some case studies that highlight their transformative capabilities:
Case Study 1: Healthcare Imaging
A leading healthcare provider utilized a data labeling platform to annotate thousands of medical images for a diagnostic AI model. With expert annotators, they achieved high accuracy in identifying anomalies, significantly improving diagnostic capabilities and operational efficiency.
Case Study 2: Autonomous Vehicles
In the autonomous vehicle industry, a prominent player employed a data labeling platform to label vast amounts of driving data for object detection. This platform allowed them to annotate images in near real-time, facilitating rapid testing and deployment of sophisticated self-driving algorithms.
Case Study 3: E-Commerce Recommendation Systems
An e-commerce giant adopted a data labeling solution to enhance product recommendation algorithms. By accurately labeling customer reviews and product attributes, they significantly improved their personalization efforts, resulting in higher customer satisfaction and increased sales.
The Future of Data Labeling Platforms
The future of data labeling platforms looks promising with advancements in technology. Here are some trends expected to shape their evolution:
1. Automation through AI
With rapid advancements in AI technologies, we can expect more platforms to incorporate AI-driven tools to automate parts of the labeling process, reducing manual effort and increasing efficiency.
2. Enhanced Collaboration Features
As remote work becomes more prevalent, data labeling platforms will likely enhance their collaboration features, allowing teams to work seamlessly from anywhere in the world.
3. Increased Focus on Security
As data privacy concerns continue to grow, future platforms will prioritize secure data handling processes, ensuring that sensitive data remains protected throughout the labeling process.
4. Expansion of Industry-Specific Solutions
We can expect platforms to offer more tailored solutions designed for specific industries, focusing on unique challenges and requirements in fields such as finance, healthcare, and manufacturing.
Conclusion: Empowering AI with Quality Data
In conclusion, a data labeling platform is indispensable for organizations looking to harness the full potential of AI and ML. By streamlining the data annotation process, these platforms provide the infrastructure necessary to ensure that high-quality labeled data is readily available for training powerful AI models. With ongoing advancements and the increasing demand for machine learning solutions, investing in a robust data labeling platform—such as those offered by Keylabs.ai—is not just beneficial; it is crucial for staying competitive in today’s data-driven landscape.